Vision Loss and V1
101 V1 receptive fields
The first thing everyone learns about V1 neurons is that they are selective for orientation — this is the first thing that was discovered by Hubel and Wiesel as they were studying cat V1 in the 1960s. The figure below has a simplified wiring diagram showing how spatially selective connections between orientation-insensitive LGN neurons and V1 neurons can create basic orientation-selective units. Near the fovea, the visual space over which V1 neurons pool inputs will be very small; V1 neurons with receptive fields at peripheral locations will sample over a larger pool of LGN inputs.
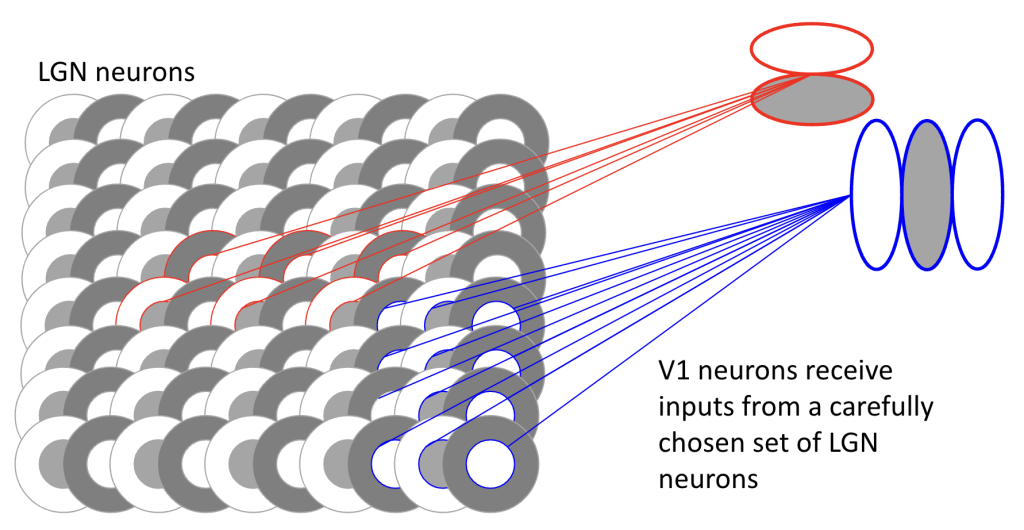
Naturally, V1 neurons do more than just signal the orientation of local features. When pooling over LGN inputs, a V1 neuron might sample from different color contrasts, so some V1 neurons are selective for color while others are just selective for luminance (brightness, independent of color).
Like the retina, V1 neural networks are built in layers, with different layers having different computational roles. The cortex is between 2.5 mm and 3 mm thick, and has somewhere between 10,000 and 40,000 neurons per cubic millimeter. So in a ~1 x 1 x 3 mm area of cortex that represents a single location in the visual field (each hemisphere of human V1 typically takes up a surface area of 12-15 cm2 to represent the entire visual field [Benson et al, 2022]), there are tens of thousands of neurons encoding many different aspects of what is going on at that one location in the visual field.
The simplified illustration above illustrates what might be happening in the input layers, which happen to be in the middle of the cortical thickness. Outputs from V1 leave from the superficial layers (away from the white matter, toward the surface of the brain). Neurons in those output layers have another opportunity to mix and match information from the middle layers to create new information. For example, in the middle layers, neurons selectively get information from just one eye or the other. Neurons in superficial layers can combine that information to compute disparity, which is an important clue about how far away an object is from the observer. Disparity as an important visual depth cue will be covered in later sections of this textbook.
One more computation that is known to happen in V1 is direction. A vertical edge formed by the mast of a sailboat, for example, might be moving to the left or the right — not every V1 neuron that is tuned to vertical orientations will respond equally well to either direction (some do — those have a low direction selectivity index, even though they might have a high orientation selectivity index). Direction can be computed either by neurons that sample selectively from LGN neurons with different response speeds at different locations (Chariker et al., 2022), or by using axon length to delay inputs to superficial layers (Conway et al., 2005), or by differences in the excitatory and inhibitory synapses in V1 (Freeman, 2021) — this is an active area of research!
References
Benson, N. C., Yoon, J. M., Forenzo, D., Engel, S. A., Kay, K. N., & Winawer, J. (2022). Variability of the surface area of the V1, V2, and V3 maps in a large sample of human observers. Journal of Neuroscience, 42(46), 8629-8646.
Conway, B. R., Kitaoka, A., Yazdanbakhsh, A., Pack, C. C., & Livingstone, M. S. (2005). Neural basis for a powerful static motion illusion. Journal of Neuroscience, 25(23), 5651-5656.
Chariker, L., Shapley, R., Hawken, M., & Young, L. S. (2022). A computational model of direction selectivity in Macaque V1 cortex based on dynamic differences between ON and OFF pathways. Journal of Neuroscience, 42(16), 3365-3380.
Freeman, A. W. (2021). A model for the origin of motion direction selectivity in visual cortex. Journal of Neuroscience, 41(1), 89-102.